|
You have four weeks’ worth of data with your evergreen ad copy. You then pause those variants and launch cost-focused copy for four weeks then compare. This method of testing can be useful and can yield good results. It’s easy to implement and only requires you to monitor your campaign for large swings in performance. The downside is that the variants never overlap with each other. Was there some seasonal effect that took place in the second weeks? Were you short on budget for the month and needed to pull back on spending to hit your levels? Did a news story impact performance for worse (or better) during either period.
It’s not perfect, but it can be useful to test sequentially to see results. Geolocation testing In a geolocation A/B testing example, you keep the existing campaign set up as it is, then create an experiment variant in a second location. This Iceland Phone Number could be either to an expanded market or a portion of where you’re currently targeting (i.e., your campaign targets the entire United States, but for this test, you make the changes only effective in a handful of states). ab testing examples - geolocation targeting ab test example screenshot To accomplish this, you need to make sure your control and experiment are mutually exclusive so there’s no overlap.
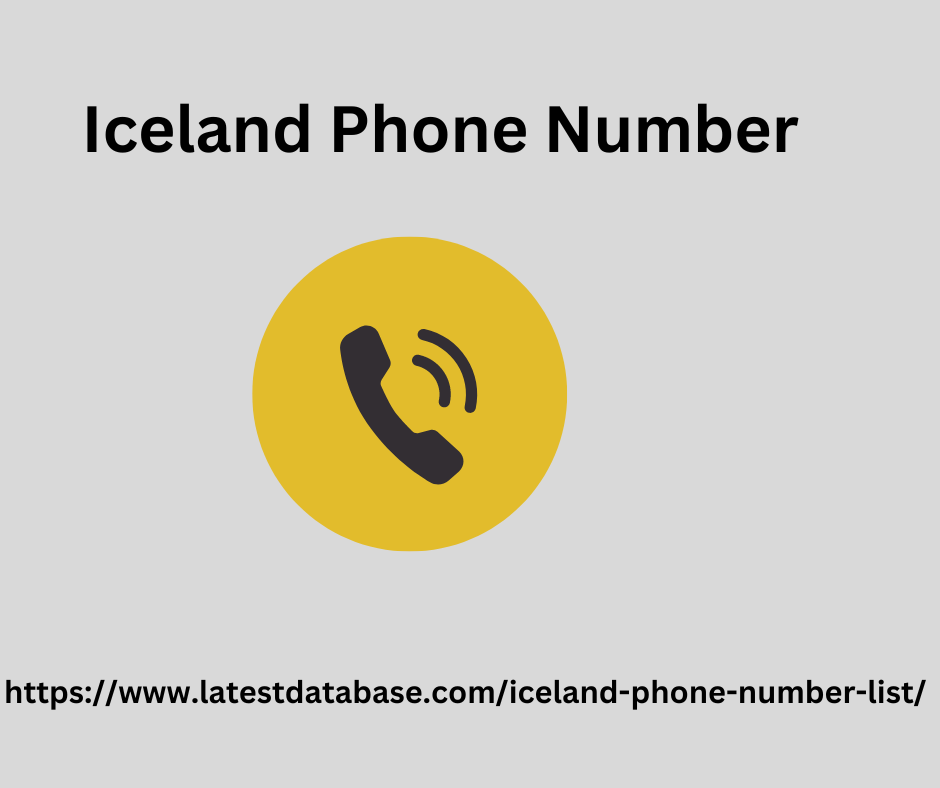
This can be done by setting up new campaigns and excluding locations in your control campaign. Unlike the sequential A/B testing example, Geolocation testing can allow you to run your variants at the same time and compare results. Any head or tailwinds you feel during the run of the test should be equal for both locations. The downfalls come when you realize that no two regions are exactly alike. Who’s to say why a cost-focused message might work better in Oklahoma than in Nebraska? Or why the East Coast performs better with automated bidding than the Mountain time zone? A/B split testing Split tests are likely the best example of A/B testing as it removes some of the cons we
|
|